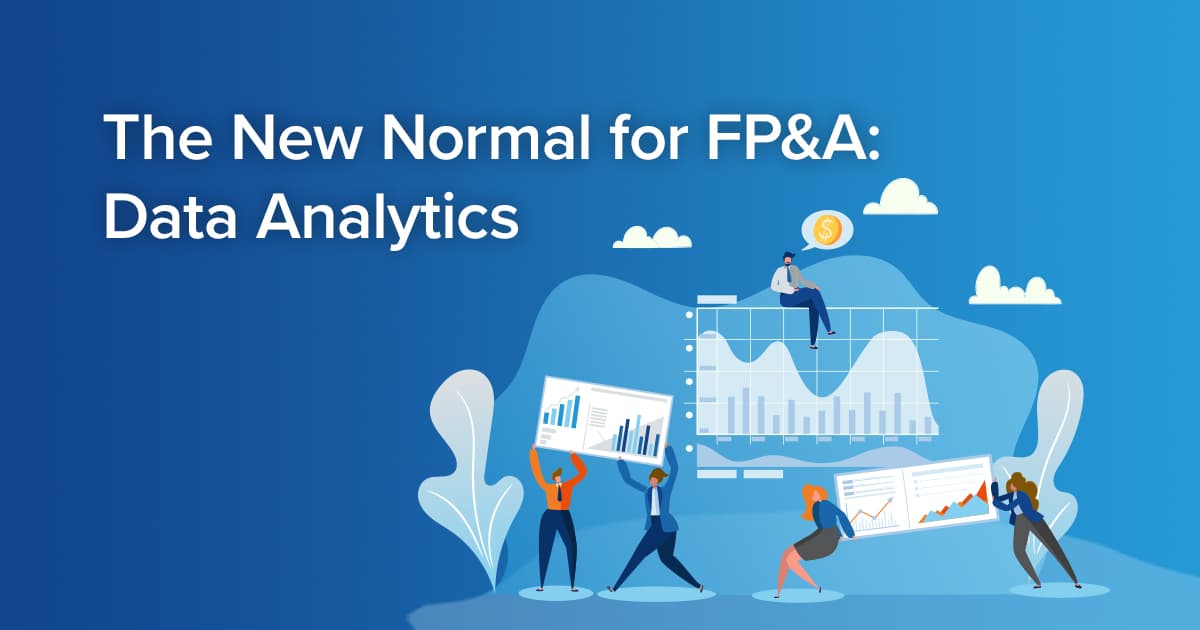
The New Normal for FP&A: Data Analytics
The term “data analytics” refers to the process of examining datasets to draw conclusions about the information they contain. Data analysis techniques enhance the ability to take raw data and uncover patterns to extract valuable insights from it.
Data analytics is the critical first step to take as we acquire data, convert that data into insights, transform those insights into knowledge and lever that knowledge to assist the organization to make those better, faster, smarter business decisions that are always important, but have an even greater priority given the tumultuous times the world currently faces.
Data analytics is not new. Today, though, the growing volume of data (currently measured in brontobytes = 10^27th power) and the advanced technologies available mean you can get much deeper insights much faster than you could in the past. The insights that data and modern technologies make possible are much more accurate and detailed, whether the data is structured/unstructured or internal/external. In addition to using data to inform your future decisions, you can also use current data to make immediate decisions.
Some of the technologies that make modern data analytics so much more powerful than they used to be include data management, data mining, predictive analytics, machine learning and artificial intelligence. While data analytics can provide many benefits to organizations that use it, it’s not without its challenges. One of the biggest challenges is collecting the data. There is much data that organizations could potentially collect, and they need to determine what to prioritize.
This challenge highlights the fact we have won the “data acquisition war.” In the not-too-distant past, a completely acceptable answer to a business question was “We don’t know.” This is because the data needed to answer the question was a) physically unavailable, b) too costly to acquire c) outdated by the time it was acquired, or d) some or all of the above.
In 2020, I would argue that data is basically free, unlimited, and immediate. It’s now a challenge of managing the massive amount of data we have access to. Gartner defines “dark data” as the data organizations collect, process, and store during regular business activities, but doesn’t use any further. Gartner also estimates 80% of all data is “dark”, while 93% of unstructured data is “dark.”
Other challenges to data analytics include data storage, data quality, and a lack of knowledge and tools necessary to make sense of the data and generate those critical insights.
In working with clients, these are some of the most common “pain points” I routinely address:
- Difficulty in extracting data out of legacy systems
- Limited real-time analytics and visuals
- Limited self-service reporting across the enterprise
- Inability to get data quickly
- Data accuracy concerns
- More time spent accessing data vs. making data-driven decisions
- The lack of integration among multiple data sources
- Historical data compatibility with the current environment (>20 years data)
- Limited internal resources
The goal in addressing these pain points is to empower your stakeholders (both within Finance/FP&A and your business partners) to be able to deliver:
- Consistent reporting and dashboards
- Self-service reporting
- Drill-down capability
- Exception reporting
The expected outcome is that users across the enterprise will have better speed, accuracy, consistency, time-savings, and flexibility. When I dive a little deeper into the specific challenges as they relate to data, the most common responses are:
- Too much ETL (Extract-Transform-Load)
- Slow refresh times
- Poor query responses
- No actionable insights
- No self-service
What is promising about addressing the challenges that have been exacerbated by the global crisis is that all of these data “pain points” and can be overcome by leveraging existing technology with the proper people, processes, and corporate culture. Too often, Finance & FP&A has been constrained by the data architecture our organizations have built (or maybe not built) over the past twenty or so years.
Typically, we take our multiple data sources and perform some level of ETL on the data. That transformed data is then stored in a data warehouse or data lake, where it can be transformed further or left in a data mart to be delivered to our reporting, business intelligence and data visualization tools as necessary. The other common data flow is taking our multiple data sources and immediately performing a high level of “spreadsheet gymnastics” in order to transform the data into our reporting tools.
The good news is that harnessing the power of existing technology available today can very easily address most common data “pain-points” and challenges – Direct Data Mapping.